By Liz Allen
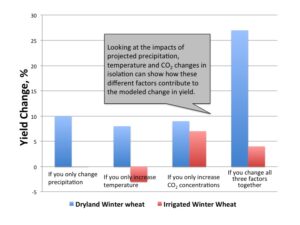
When I was a Masters student in central Massachusetts I spent my nights and weekends bartending at a pub that catered to an eclectic mixture of college students, blue-collar workers and retirees from the neighborhood. Working there, I had a lot of conversations with regulars about my research and for the most part they were interested in discussing what scientists are doing to understand and address climate change impacts. When I started telling everyone that I was about to move to Washington State University to work on my PhD as part of a regional climate modeling research team, a typical response was, “Modeling? What kind of magazine hires scientists to be models? Har har har.” Jokes about my future in the high fashion modeling industry aside, the truth is that most people have very limited exposure to what a climate model is, what actual data it’s based on, and how it might be used.
Even if you are better versed in climate science than the regulars at the bar where I worked, chances are that you are less familiar with modeling than you are with experimental science that happens in the laboratory or field. The skepticism that many people have about environmental modeling may be rooted in the often-made observation that weather forecasts and economic projections are “frequently wrong”. Some individuals view modeling with suspicion because of the potential for model outputs to suggest a need to change practices. However, it is only through modeling that future conditions can be studied—by making projections based on our understanding of the underlying processes at work and an assessment of likely future trends. Assessing change over long time periods or across large areas may be prohibitively expensive or logistically impractical to study with experimental methods alone. In this first installment of the series I’ll discuss what makes modeling distinct from other forms of scientific inquiry and why we need models to study climate change.
Models are, by definition, simplifications of real-world systems and processes. By identifying what factors drive change and what feedback loops exist and then representing them mathematically we can build a more complete understanding of how complex environmental systems work. For example, if we suspect that winter wheat yields in Pullman, Washington depend on the length of the growing season, precipitation, temperature and even the concentration of carbon dioxide (CO2) in the surrounding air, we can run a crop model with real observed values for growing season, precipitation, temperature and CO2, and compare the yield projections our model makes with actual observed crop yields to assess whether we have captured key driving factors or not. If our model consistently and effectively produces yields that are close to the real values, we can then use it to predict crop yields for that location under other climate conditions with some confidence.
Once a model has been validated in this way, we can use it in other ways too. For example, we can isolate specific processes and feedbacks in order to explore their role in the system as a whole. This can be particularly useful when those drivers are tightly interrelated, as with climate change: increases in CO2 concentrations drive changes in temperature in precipitation, so it’s hard to work out which factors are the ones the crop is really responding to. Researchers studying climate change impacts on winter wheat in the Columbia River Basin used a crop model to look at how temperature, precipitation and CO2 concentrations contribute to yield. They found that under climate change, dryland winter wheat yields would increase thanks to an earlier start of the growing season and more early season precipitation. In contrast, irrigated winter wheat yields would not change drastically: projected changes in precipitation would have little impact for irrigated crops, and the decrease in yields due to higher temperature would be counterbalanced by the increase in yields due to increased CO2 concentrations.
One fascinating aspect of modeling is that it opens up the opportunity to play out different “what if” scenarios without facing the consequences that those decisions and events would have in the real world. This means that we can explore what changes in climate might mean for specific crops in the region and what the impacts of specific management practices might be, such as planting different crop varieties or shifting planting and harvesting dates. This scenarios-based approach allows us to ask the following kinds of questions: If you were to plant winter wheat variety “x” instead of variety “y”, would your yields change under projected shifts in climate? How would regulations about fertilizer and pesticide application affect yields? If there are likely future situations in which our current management and policy strategies don’t perform well, what can be done to minimize negative impacts?
Liz Allen is a graduate research assistant in the School of the Environment at Washington State University where she is a member of the BioEarth Regional Earth Systems Modeling team.
Comments