By Liz Allen
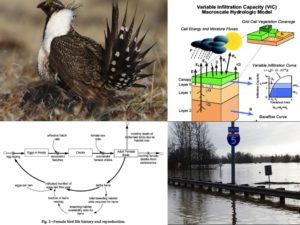
Within my research team, I have the very unusual role of studying my fellow scientists. I use research methods from anthropology and sociology—interviews, surveys and participant observation—to understand how academic scientists think about the relationship between their research and their stakeholders, who make management and policy decisions about water resources, forests and agricultural systems. It’s been eye opening to realize that environmental modelers trained in different disciplines often have different ideas about what modeling is and what models are for. As I learned that scientists think about models in diverse ways, I was able to recognize the limitations and strengths of different modeling approaches. I also gained a deeper appreciation of just how challenging—and valuable—interdisciplinary collaboration can be.
Broadly speaking, the scientists I studied fell into two different camps, depending on the approach they take to modeling complex environmental systems. On the one hand you have the big-picture modelers, who take a “top-down” approach, and on the other you have the detail-oriented modelers, who favor a “bottom-up” approach. Both approaches use mathematical equations to describe real-world phenomena, but underlying views of how a model helps solve a problem differ. So understanding these two different approaches to modeling can be helpful in interpreting model projections and sources of uncertainty.
Let’s start with the big-picture modelers, called system dynamics modelers. In their top-down approach, they begin by thinking about a thorny problem that modeling might shed light on. This is typically an issue of broad social relevance you would read about in the news, such as the possibility of sage grouse being listed as an endangered species, which would drastically impact agricultural operations. Washington State University scientists worked with the Foster Creek Conservation District to develop a model that explores how land use decisions interact with sage grouse populations (Beall and Zeoli, 2008). Their model relates the proportion of land converted from wheat cropland back to shrub-steppe habitat to the projected numbers of nesting sage grouse hens and surviving chicks, and then assesses the economic impacts of that conversion for landowners. Once causal relationships have been diagrammed, specific quantitative data can be integrated into the system dynamics model, enabling the identification of “tipping points” in the system. In our sage grouse example, the researchers found that a viable Douglas county population cannot be sustained if the chick survival rate drops below about 13% in any year. .
Often, system dynamics models are developed in close collaboration with stakeholders—such as the Conservation District, in the sage grouse habitat modeling example—to reach new understanding about a resource management concern. This modeling approach can be tremendously useful in bringing different perspectives about system relationships to the table, and enabling managers to consider the possible consequences of their decisions. That’s why this modeling approach is most appropriate when the goal is to understand how complex social and environmental systems interact. Top-down system dynamics models, however, cannot generally be used to forecast future conditions.
That’s where the detail-oriented “bottom-up” modeling approach comes in. These models are sometimes called process-based models or “engineering models” and are developed by mathematically representing system components and their interactions at the most elemental level. Process-based modelers describe basic processes, and then tie together these elemental processes across time and space to create a mathematical representation of an interrelated system with feedbacks between processes.
Clear as mud? Here’s an example: the VIC (Variable Infiltration Capacity) model, which was developed at the University of Washington, simulates full water and energy balances and has been adapted for use in most major river basins around the world (Liang et al. 1994). Input data needed to run the VIC model include daily meteorological data, and data on how the vegetation—root depth and plant canopy characteristics—and soil type vary across space. A molecular-level understanding of how water infiltrates soil and is taken up by plants moment-by-moment is scaled up to represent the fate of water in the river basin over long periods of time.
As you might imagine, process based models take tremendous computing power to run. These models are based on massive amounts of spatially-explicit data. The coding language and mathematical relationships used to create process-based models may be very difficult for non-modelers to understand. However, because these models represent processes at a fine spatial and temporal scale, bottom-up models are key to our understanding of how changes in temperature and precipitation patterns might affect streamflow and plant growth, for example. And it is this understanding that allows us to make projections about the future, such as under what conditions flooding might occur.
So what good is this distinction between top-down and bottom-up models to you? Mainly because the type of models that provide information about climate change impacts on agriculture are built by uniting top-down and bottom-up perspectives. Stay tuned for Part 3 of this series, where I’ll delve into the details of integrating those two approaches, and what happens when scientists do that.
For now, though, I’m interested in what questions you, as an agriculturally-minded reader, have about environmental models? Are you trying to interpret the results of a particular model? Or evaluating a series of models to see which best fits your needs? Want to know what those models can and cannot project, or what data they are based on? Do you have questions about the assumptions embedded in existing climate impacts models? Post a comment below, and we’ll keep the conversation going (and give me more fodder for research at the same time)!
References:
Beall, A., & Zeoli, L. (2008). Participatory modeling of endangered wildlife systems: Simulating the sage-grouse and land use in Central Washington. Ecological Economics, 68(1), 24-33.
Liang, X., D. P. Lettenmaier, E. F. Wood, and S. J. Burges, 1994: A Simple hydrologically Based Model of Land Surface Water and Energy Fluxes for GSMs, J. Geophys. Res., 99(D7), 14,415-14,428.
Liz Allen is a graduate research assistant in the School of the Environment at Washington State University where she is a member of the BioEarth Regional Earth Systems Modeling team.
Comments