By CIRCulator Editorial Staff
Reprinted from: The Climate CIRCulator
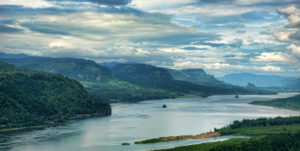
MANY CLIMATE ADAPTATION plans are largely based on climate model projections of precipitation. However, many of these models are notorious for their inability to accurately simulate seasonal rainfall at the regional level. This is especially true for the Pacific Northwest where models consistently depict summers as being much wetter than observed when they simulate past climate.
Researchers Mehmet Demirel and Hamid Moradkhani address this issue in their recent paper in Climatic Change. The paper focuses on the Columbia River Basin. Global climate models (GCMs) routinely simulate this region being wet throughout the year, essentially modeling a continuous drizzle in the basin that persists into the normally dry summer months. As the Columbia River is the largest source of freshwater in the Pacific Northwest—supplying drinking water, irrigation, ecosystem benefits, energy, and recreation for some 11 million inhabitants—using these models to help us anticipate the effects of climate change requires artfully dealing with their shortcomings.
In their paper Demirel and Moradkhani assessed this ‘drizzle effect’ and found that GCMs capture the summer dry season quite well in coastal regions of Oregon and Washington. But inland, over eastern Washington, Idaho, and British Columbia, GCMs aren’t capturing the seasonality of precipitation, showing instead a constant drizzle nearly all year long. The seasonality is especially poor in the high desert regions of south central Idaho and eastern Oregon.
In their study, Demirel and Moradkhani developed a technique to help make up for the GCMs precipitation shortcomings. To do this the researchers employed an advanced statistical technique to average over an ensemble of GCMs, giving the best models the most weight. This methodology allowed the researchers to improve the quantity of annual precipitation simulated by 15 to 20 percent. Unfortunately, the drizzle effect was still present.
To improve precipitation, the researchers employed another step: downscaling.
As the “global” in global climate models implies, GCMs have been designed to simulate the big (global) climate picture. This is fine if you’re interested in global trends. The problem comes when you try to translate those trends to the regional level. The solution climate researchers have come up with is called downscaling.
Think of GCMs as large video games made of square cells, with each cell representing a section of the earth. GCMs vary, but most grid cells have a resolution of about 112 km (roughly 70 miles) to a side. Downscaling increases this resolution to a more practical, regional scale.
In their study Demirel and Moradkhani used downscaled climate data with a resolution of 6 km x 6 km (a little less than 4 miles by 4 miles). Downscaling adds detail to the models by incorporating topography and historical relationships with larger scale quantities. In general, this makes downscaled models a better statistical fit for a given region’s climate. But do these downscaling techniques also improve the ability to capture the seasonality of precipitation? This is the question Demirel and Moradkhani set out to answer with their model averaging technique.
Demirel and Moradkhani employed ten GCMs and two different downscaling techniques: Bias-Correction Spatial Disaggregation (BCSD) and Multivariate Adaptive Constructed Analogs (MACA). (MACA is the brainchild of CIRC researcher John Abatzoglou.) Their study compared daily precipitation from each downscaled GCM to the observed precipitation from 1970 to 1999 in the Columbia River Basin.
When a single GCM is downscaled and compared to historical observations, it may represent the past accurately in some regions but not so well in others. This makes it difficult to choose which GCM to use when making management decisions. Taking the average of several GCMs improves the accuracy; however, when this is done, each model usually receives the same amount of weight. This means that the worst model and the best model contribute equally to the average. Similar to T-ball, everyone gets the same amount of playing time regardless of his or her skill level.
Demirel and Moradkhani decided that the future of freshwater resources in the Northwest is too important for equal playing time. They employed a statistical technique called Bayesian model averaging to ensure that the best performing models were given the most weight, essentially playing the best players in a sort of championship.
This technique compared precipitation from each downscaled GCM to the observed precipitation at every grid cell, and then gave the most weight to the consistently accurate models. For example, say one GCM simulates precipitation well near the coast but is always too wet in the mountains. This GCM would be given more weight near the coast and less weight in inland mountainous regions.
The weighted model average simulated the quantity of annual precipitation received in the Columbia River Basin 15 to 20 percent more accurately than any individual downscaled GCM. However, the weighted ensemble still didn’t capture the summer dry season, especially in the high desert regions of eastern Oregon and south central Idaho.
Demirel and Moradkhani’s results illuminate one of the major limitations of future climate model projections. The lack of seasonality hinders the GCMs ability to capture important characteristics of precipitation. In some watersheds the timing of precipitation can be more important than the amount received. The implication is if climate models aren’t capturing long dry spells, resource managers can’t anticipate the potential effects of climate change. There is an urgent need for improving modeled precipitation; the effectiveness of climate adaptation efforts depends on it.
Editorial Note: The authors do not state whether their model averaging technique improved upon the regular ensemble average. Comparisons were only made with individual GCMs.
Reference:
Demirel, Mehmet C., and Hamid Moradkhani. “Assessing the impact of CMIP5 climate multi-modeling on estimating the precipitation seasonality and timing.” Climatic Change (2015): 1-16. Online Access
Comments